Evolution of AI Model Architectures and Breakthrough Technologies
More Efficient Architecture Models
The future of conversational artificial intelligence is intrinsically linked to the evolution of AI model architectures, which are undergoing rapid transformation towards higher efficiency and performance. A key aspect of this evolution is the Mixture-of-Experts (MoE) technology, representing a significant shift from monolithic models to a modular approach. This architecture divides the neural network into specialized "experts" that are activated only for specific types of inputs, dramatically increasing computational efficiency.
A parallel trend is the implementation of sparse activation mechanisms, which allow selective activation of only relevant parts of the model for a specific task. Unlike traditional architectures where all neurons are activated, sparse activation dramatically reduces computational demands and enables the construction of significantly larger models while maintaining efficient inference time. The practical consequence of these innovations is the possibility of deploying more sophisticated AI chatbots even on edge devices with limited computational capacities.
Specialized Modular Architectures
Another direction of development involves specialized modular architectures, which combine generic components with domain-specific modules. These systems integrate pre-trained generic foundations with narrowly specialized modules for specific domains like medicine, law, or finance, enabling expert-level knowledge and capabilities without needing to train the entire model from scratch. This approach significantly reduces development costs while increasing the accuracy and relevance of responses in specific fields.
Expansion of Contextual Understanding
The evolution of AI model architecture is heading towards a radical expansion of the context window, representing a fundamental shift in the ability to process and coherently respond to complex inputs. Current limitations in the range of tens or hundreds of thousands of tokens will be surpassed in upcoming model generations, moving towards millions of tokens or potentially practically unlimited context. This expansion will enable conversational systems to maintain consistent long-term interactions and process extensive documents like complete books, research papers, or technical manuals in a single pass.
Technological enablers of this transformation include hierarchical context processing, where the model operates with multi-level representations – from detailed local levels to global abstractions. Another innovative approach is recursive summarization, where the system continuously compresses historical information into dense representations that preserve key information while minimizing memory requirements. An emerging technique is also attention caching, which optimizes repeated computations on overlapping parts of the context.
Dynamic Context Management
Advanced architectures implement dynamic context management, which intelligently prioritizes and selects relevant information based on its importance to the current conversation. This approach combines strategies like information retrieval, local cache, and long-term memory storage for efficient handling of practically unlimited amounts of contextual information. The practical impact of these innovations is the ability of AI assistants to provide consistent, contextually relevant responses even within complex, multi-session interactions occurring over a longer time horizon.
Advanced Cognitive Abilities
A fundamental trend in the evolution of AI architectures is the shift from purely reactive systems to models with advanced cognitive abilities, which qualitatively transform their utility in solving complex problems. The new generation of conversational systems demonstrates significantly more sophisticated causal reasoning – the ability to identify causal relationships, distinguish correlation from causation, and construct robust mental models of problem domains. This capability allows AI chatbots to provide deeper analyses, more accurate predictions, and more valuable data interpretations compared to previous generations.
A parallel development direction is progress in abstract and analogical thinking, where models can identify high-level patterns and apply concepts from one domain to problems in another. This ability is key for creative problem-solving, interdisciplinary knowledge transfer, and identifying non-obvious connections that often represent the highest value in complex decision-making. A significant dimension is also the development of meta-cognitive abilities – the model's capacity to reflect on its own thought processes, evaluate the quality of its responses, and identify the limits of its own knowledge.
Algorithmic Reasoning and Multi-Step Problem Solving
Advanced architectures demonstrate significant progress in algorithmic reasoning and multi-step problem solving – the ability to decompose complex problems into a series of sub-steps, systematically solve them, and integrate partial results into a coherent solution. This capability is essential for tasks requiring a structured approach, such as mathematical derivations, complex planning, or debugging complex systems. Combined with increased accuracy (the ability to minimize hallucinations and factual errors), these advanced cognitive capacities transform AI chats from primarily communication tools into sophisticated cognitive assistants capable of providing substantive support in solving real-world problems.
This is ultimately visible in "thinking" models today. For example, Gemini 2.0, Claude 3.7 Sonnet, or ChatGPT o1 have these features. Take a look at the example we created for you using the o3-mini model, which has a similar thinking mechanism.
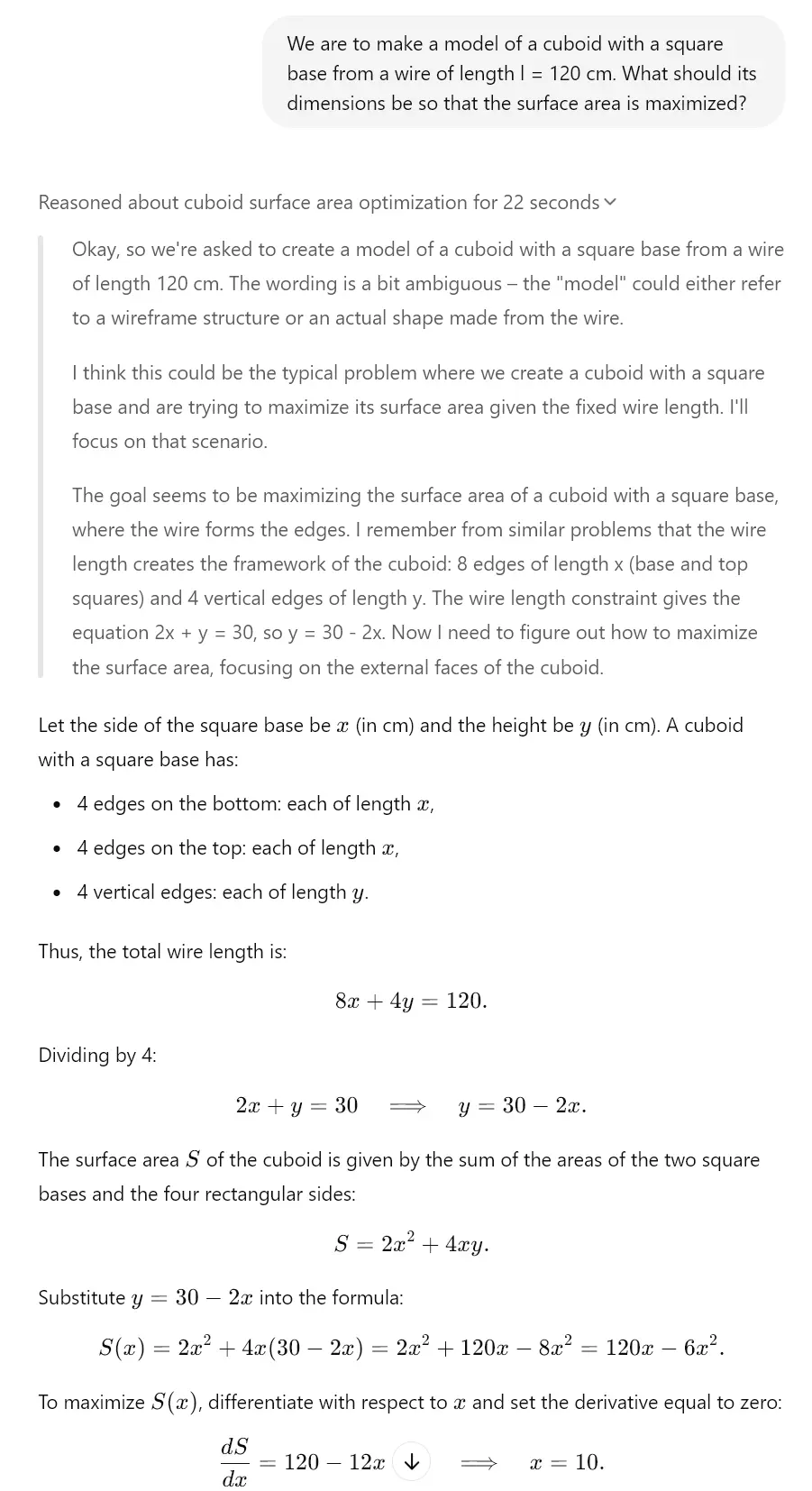
Optimization of Parameters and Outputs
A critical aspect of the evolution of AI architectures is the continuous optimization of parameters and outputs, which increases the efficiency and quality of generated content. Quantization techniques represent a significant advancement in model compression and involve methods for reducing memory and computational requirements while preserving most of the original performance. Modern approaches like post-training quantization and mixed-precision inference allow reducing model size by up to 75% with minimal performance degradation, dramatically expanding the range of devices capable of hosting sophisticated conversational AI systems.
A parallel trend is optimization through knowledge distillation, where knowledge from large "teacher" models is transferred to more compact "student" models. This process effectively compresses information captured in complex neural networks into smaller architectures that can be deployed in resource-constrained environments. Significant potential also lies in hardware-specific optimizations, where the model architecture is specifically tailored for maximum performance on particular hardware (CPU, GPU, TPU, neuromorphic chips), enabling significantly higher inference speeds.
Adaptive Output Mechanisms
Advanced architectures implement adaptive output mechanisms that dynamically adjust response generation based on context, accuracy requirements, and available computational resources. These systems intelligently balance quality, speed, and efficiency through techniques like early-exit inference and progressive rendering. The practical consequence of these optimizations is the ability to deploy highly sophisticated AI assistants even in edge computing scenarios such as mobility, IoT devices, or wearable augmented reality devices, where traditional large language models are unusable due to resource constraints.
Neural Networks and Their Development
A fundamental aspect of AI model evolution is innovation in neural network architecture, which defines their capabilities and limitations. Hybrid architectures combining different types of neural networks to maximize their strengths hold transformative potential. These systems integrate transformer-based models optimized for text understanding with convolutional networks for visual analysis, recurrent networks for sequential data, and graph neural networks for structured information, enabling the creation of versatile systems capable of operating across various domains and data types.
Another development direction involves recurrent transformers, which address the limitations of standard transformer architectures in sequential processing and temporal reasoning. These models implement recurrent mechanisms like state tracking and iterative refinement, significantly improving their ability to model dynamic processes, gradual reasoning, and complex sequential dependencies. This capability is essential for tasks such as simulation, strategic planning, or long-term prediction, which require sophisticated understanding of temporal relationships.
Self-Modifying and Self-Improving Architectures
An emerging trend is represented by self-modifying and self-improving architectures, which can adapt their structure and parameters in response to specific tasks. These systems implement meta-learning mechanisms that continuously optimize their internal configuration based on feedback loops and performance metrics. A key dimension is also Neural Architecture Search (NAS), where AI systems automatically design and optimize new neural network architectures specifically tailored to particular use cases. This approach accelerates the iteration of AI models and enables the creation of highly efficient custom architectures for specific application domains of conversational AI.
Impact of Evolution on Conversational AI
The overall impact of the evolution of AI architectures on conversational systems is transformative, bringing a fundamental shift in their capabilities and application potential. Multimodal integration is a key element of this transformation – modern architectures allow seamless transition between text, image, sound, and other modalities, expanding conversational interfaces beyond purely text-based interaction. This integration enables AI chatbots to analyze visual inputs, respond to multimedia content, and generate rich media responses combining text with visual or auditory elements. For a more detailed look at this topic, you can refer to the analysis of autonomous AI agents and multimodal systems.
A parallel aspect is continuous real-time learning, where advanced architectures can continuously update their knowledge and adapt to new information without requiring complete retraining. This approach addresses a key limitation of traditional static models – the rapid obsolescence of knowledge in dynamically evolving domains. An emerging architectural approach is also local fine-tuning, which optimizes model performance for a specific context or user while preserving the generic capabilities of the base model.
New Generation of Conversational Assistants
The cumulative effect of these architectural innovations is the emergence of a new generation of conversational assistants with qualitatively different capabilities. These systems transcend the paradigm of reactive question-and-answer tools towards proactive cognitive partners capable of independent reasoning, continuous learning, and adaptation to specific user needs. Practical applications include personalized educational systems dynamically adapting content and pedagogical approach to the student's learning style; research assistants capable of formulating hypotheses and designing experiments; or strategic advisors providing substantive support in complex business decision-making. This evolution represents a significant shift towards AI systems that function as true cognitive amplifiers, exponentially expanding human cognitive capacities.